In modern technology, the convergence of Artificial Intelligence (AI) and Computer Vision has catalyzed groundbreaking advancements that have forever transformed our world. The journey of these interconnected fields, from their humble origins to their contemporary prowess, is a testament to human ingenuity and relentless innovation. This narrative embarks on a captivating exploration of the intertwined histories of AI, Computer Vision, and AI Image Recognition, with a particular focus on the pivotal role played by DARPA.
AI, as a concept, has fascinated researchers and scientists since its formal inception in the 1950s. It symbolizes the endeavor to bestow machines with human-like cognitive abilities, including the capacity to perceive and interpret visual data—an aspiration at the core of Computer Vision. In its early stages, Computer Vision primarily concentrated on rudimentary image processing tasks, such as edge detection and pattern recognition. These foundational efforts laid the groundwork for the more sophisticated AI-driven systems we have today.
The 1980s witnessed a significant turning point when government agencies like DARPA invested heavily in Computer Vision research. DARPA’s involvement fueled pioneering investigations into object recognition and scene understanding, propelling the field forward. However, it wasn’t until the late 20th century that machine learning techniques, particularly neural networks, began to gain prominence, revolutionizing the capabilities of Computer Vision. This newfound power manifested itself prominently in AI Image Recognition.
The 2010s ushered in the Deep Learning revolution, characterized by the advent of Convolutional Neural Networks (CNNs) that drastically improved image recognition accuracy. A watershed moment occurred in 2012 when a CNN-based approach triumphed at the ImageNet Large Scale Visual Recognition Challenge (ILSVRC). This victory not only showcased the prowess of AI Image Recognition but also sparked a resurgence of interest in the field.
As we delve deeper into this historical narrative, we will uncover the pivotal milestones, key breakthroughs, and transformative applications that have defined the trajectory of AI, Computer Vision, and AI Image Recognition. Our journey through time will reveal not only the remarkable progress made but also the challenges and ethical considerations that continue to shape the future of these fields.
Table of Contents
What is AI image recognition?
AI image recognition, also known as computer vision, refers to the technology and processes by which artificial intelligence systems are trained to interpret and understand visual information from images or videos. This field of AI focuses on enabling machines to recognize and make sense of the visual world, much like the human visual system.
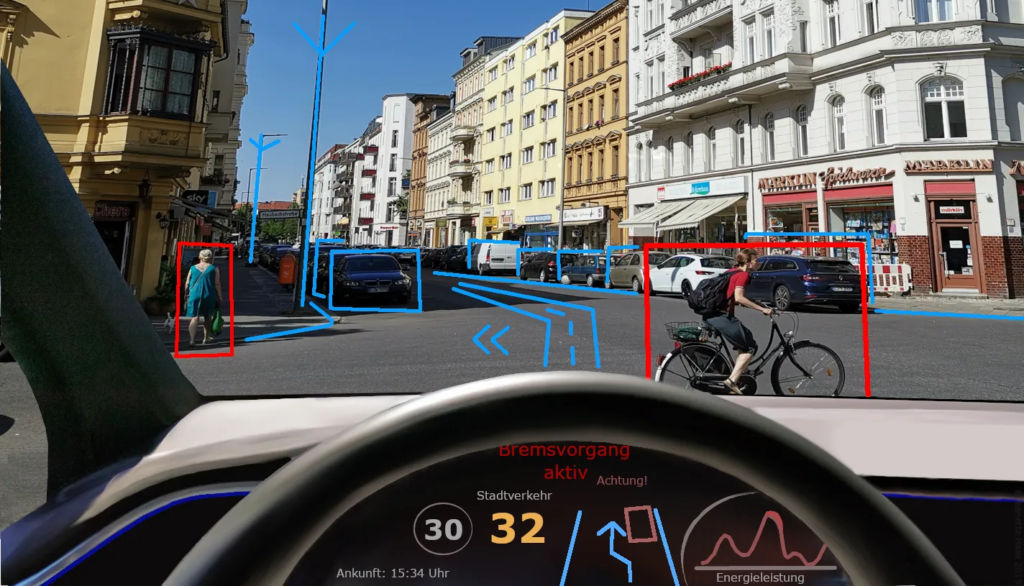
At its core, AI image recognition involves the use of advanced algorithms and deep learning techniques, such as convolutional neural networks (CNNs), to analyze and identify objects, patterns, or features within digital images or video frames. These algorithms learn from large datasets of labeled images, allowing them to extract meaningful information, detect objects, and classify them based on predefined categories or labels.
AI image recognition has a wide range of practical applications across various industries, including healthcare (diagnosing medical conditions from X-rays and MRIs), automotive (enabling autonomous vehicles to understand their surroundings), security (facial recognition and object tracking), e-commerce (product recommendation based on visual similarities), and entertainment (content tagging and video analysis).
This technology has significantly advanced over the years, achieving levels of accuracy and capability that were once considered challenging for machines. However, challenges remain, including issues related to bias in data and ethical concerns, which require ongoing research and development to ensure the responsible and fair use of AI image recognition in various applications.
What is the role of artificial intelligence and image processing in computer vision?
Artificial intelligence (AI) and image processing play crucial roles in the field of computer vision, working synergistically to enable machines to interpret and understand visual data:
Image Processing
Image processing is the foundational step in computer vision. It involves a set of techniques used to enhance, manipulate, and analyze digital images. Image processing techniques can preprocess raw visual data by filtering noise, enhancing contrast, and extracting relevant features. These processed images serve as input for subsequent AI algorithms.
Feature Extraction
AI algorithms in computer vision, such as convolutional neural networks (CNNs), use image processing techniques to extract meaningful features from images. Features could be edges, textures, shapes, or any other visual patterns that aid in object recognition and classification.
Object Detection and Recognition
AI models trained on large datasets leverage these extracted features to recognize objects within images. Through the use of machine learning, deep learning, and pattern recognition, AI algorithms can identify and categorize objects or scenes, enabling computers to understand what they “see.”
Semantic Segmentation
AI-driven image processing techniques also enable semantic segmentation, which involves pixel-level labeling of objects in an image. This precise identification of object boundaries is vital for applications like autonomous vehicles and medical image analysis.
Real-Time Processing
The integration of AI and image processing allows for real-time visual analysis, making it applicable in scenarios like robotics, surveillance, and augmented reality, where immediate decisions and actions are required based on visual data.
In essence, AI and image processing complement each other in computer vision. While image processing prepares and refines raw visual data, AI harnesses this processed information to recognize, interpret, and make decisions based on the content of images or videos. This synergy enables a wide range of applications across industries, from healthcare to autonomous systems, significantly advancing our ability to interact with and understand the visual world.
History of Computer Vision and AI Image Recognition
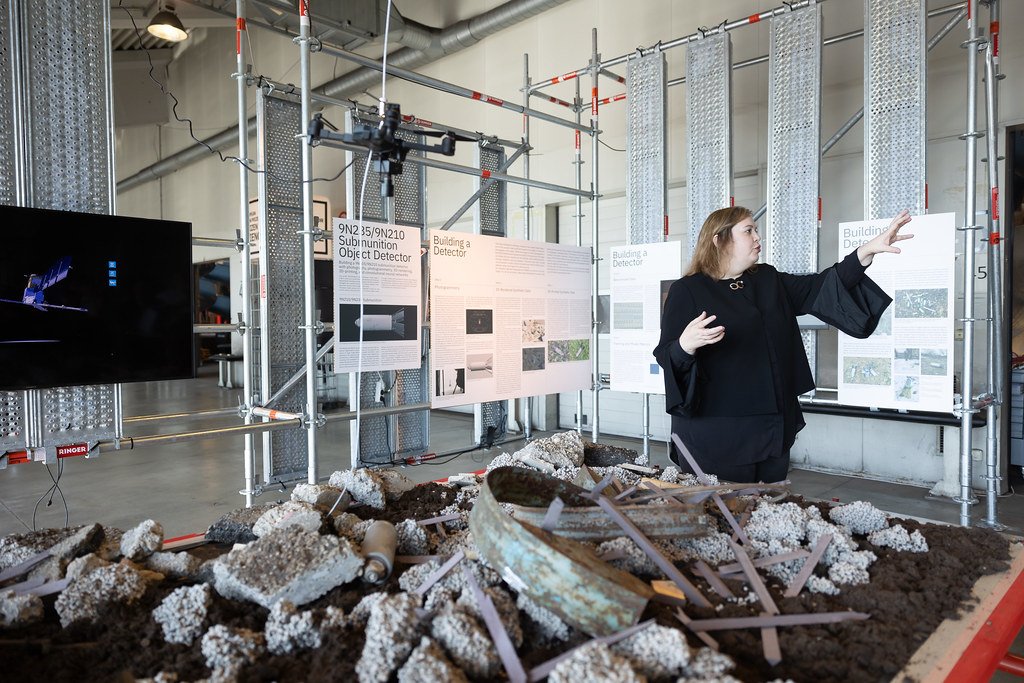
Computer Vision and AI Image Recognition have a rich history that spans several decades. Here’s a brief overview of their evolution:
Early Foundations (1950s-1960s)
The origins of computer vision and AI image recognition can be traced back to the 1950s, a time when the idea of imbuing machines with the ability to “see” and comprehend visual information was in its infancy. This era witnessed the inception of AI as a formal field of study, with a landmark event occurring in 1956 at the Dartmouth Conference. This conference, organized by John McCarthy, Marvin Minsky, Nathaniel Rochester, and Claude Shannon, marked the official founding of Artificial Intelligence (AI) as a discipline.
Within the broader realm of AI, computer vision emerged as a subset that aimed to replicate human vision and perception using computational algorithms. Early efforts in computer vision focused on fundamental image processing tasks like edge detection and basic pattern recognition. These initial endeavors laid the foundational concepts for more sophisticated techniques and technologies that would eventually lead to the development of modern AI image recognition systems. Thus, the 1950s and the Dartmouth Conference stand as pivotal moments in history, heralding the birth of AI and the initial exploration of the fascinating field of computer vision.
Initial Concepts (1960s-1970s)
In the early stages of computer vision, which emerged in the 1950s and 1960s, the focus was primarily on rudimentary image processing tasks. Researchers aimed to develop algorithms that could mimic the basic capabilities of human vision, albeit in a limited capacity. Some of the fundamental tasks included edge detection, where algorithms identified the boundaries of objects within images, and pattern recognition, which involved identifying specific patterns or shapes within visual data.
During this era, a groundbreaking project known as the General Problem Solver (GPS) emerged as a pioneering effort in computer vision. Developed by Herbert A. Simon and Allen Newell in the late 1950s, GPS was not exclusively a computer vision system but a more general-purpose artificial intelligence program. Nevertheless, it made significant contributions to the field of computer vision by demonstrating its potential to solve geometric analogy problems. GPS could analyze visual information and reason about geometric relationships, opening up new possibilities for applying AI to visual data interpretation.
These early endeavors laid the foundation for subsequent developments in computer vision and set the stage for the integration of AI techniques to address more complex visual recognition challenges in the decades that followed.
Government Research and Funding (1980s)
During the 1980s, computer vision research underwent a transformative phase, marked by substantial attention and funding from government agencies, most notably the Defense Advanced Research Projects Agency (DARPA) in the United States. DARPA recognized the strategic importance of computer vision for applications in defense and security, prompting substantial investments in research and development.
Researchers during this era began to grapple with the complex challenges of computer vision, focusing on two critical aspects: object recognition and scene understanding. However, it’s important to note that the capabilities of computer vision systems during this period were relatively limited compared to today’s standards.
In object recognition, researchers aimed to teach computers to identify and classify objects within images or video frames. While progress was made, these systems often struggled with variations in lighting, scale, and pose, making them far from perfect.
Scene understanding involved deciphering the context and relationships between objects within a scene, a task that proved even more challenging. Understanding complex scenes required addressing issues like occlusion, object interactions, and spatial relationships, all of which pushed the boundaries of technology at the time.
Nonetheless, the 1980s served as a crucial foundation for subsequent advancements in computer vision. It was during this period that researchers and agencies began to recognize the immense potential of this field, paving the way for future breakthroughs and innovations that have revolutionized the way we perceive and interact with visual data today.
Advancements in Machine Learning (1990s-2000s)
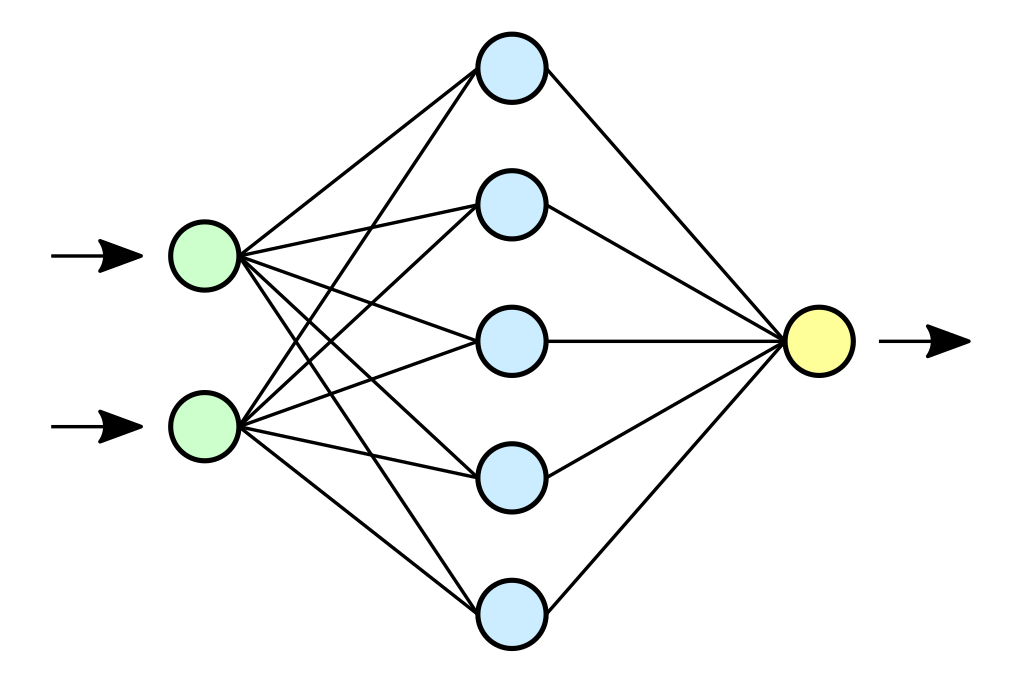
During the 1990s, computer vision experienced a notable shift with the emergence of machine learning techniques. Neural networks and support vector machines (SVMs) became prominent tools in the computer vision toolbox, signifying a departure from traditional rule-based methods. These machine-learning approaches brought a remarkable improvement in the performance of computer vision systems.
One of the pivotal applications that gained traction during this period was face recognition. Researchers started developing algorithms capable of identifying and verifying individuals based on facial features, paving the way for applications like security systems and facial authentication in today’s smartphones.
Handwriting recognition was another area of focus, where machine learning algorithms were employed to decipher handwritten text, allowing for greater automation in tasks like postal sorting and digitizing handwritten documents.
The 1990s also witnessed a significant boost in the capabilities of image recognition, thanks to the availability of large datasets and increased computational power. These factors enabled researchers to train more sophisticated models, making it possible to tackle complex tasks like object recognition and scene understanding. As a result, computer vision evolved from being a theoretical concept to a practical technology with a wide range of real-world applications.
Deep Learning Revolution (2010s-Present)
The breakthrough in deep learning, specifically with the advent of convolutional neural networks (CNNs), ushered in a transformative era for computer vision and AI image recognition. CNNs, inspired by the human visual system, brought a new level of sophistication to the field by enabling machines to learn hierarchical representations of visual data. These networks could automatically extract meaningful features from images, allowing for more accurate and robust object recognition.
A pivotal moment in this journey occurred in 2012 when a deep learning-based approach dominated the ImageNet Large Scale Visual Recognition Challenge (ILSVRC). This competition, which involved classifying millions of images into thousands of categories, had long been a benchmark for the progress of AI image recognition. The victory of a deep learning model showcased the immense potential of this technology and sparked a renewed interest in the field.
As a result, major tech giants like Google, Facebook, and Microsoft recognized the immense value of computer vision research and invested heavily in it. These investments led to the development of powerful image recognition models and the integration of computer vision into various products and services, from advanced image search to facial recognition and autonomous vehicles. The convergence of deep learning, abundant data, and substantial resources paved the way for the rapid advancement of AI image recognition, revolutionizing industries and reshaping our interaction with visual information.
Practical Applications (2010s-Present)
Computer vision and image recognition have permeated numerous industries, ushering in transformative applications and reshaping the way we interact with technology and data.
In autonomous vehicles, these technologies enable cars to perceive their surroundings, identify obstacles, and make split-second decisions to ensure safety. This not only enhances road safety but also paves the way for the realization of self-driving cars, promising more efficient transportation systems.
In healthcare, medical image analysis powered by computer vision aids in diagnosing diseases from radiological images, detecting anomalies in scans, and even assisting in surgical procedures. This has the potential to improve patient outcomes, reduce human error, and enhance the efficiency of healthcare delivery.
Retail experiences have been revolutionized by AI image recognition, with cashier-less stores offering a seamless shopping experience. Automated checkout systems utilize computer vision to track items selected by customers and charge them automatically, eliminating the need for traditional cashiers.
However, these advancements have not been without controversy. The ethical and privacy implications of AI image recognition, especially in the context of facial recognition technology, have spurred intense debates. Concerns about surveillance, discrimination, and data privacy have led to increased regulation and scrutiny by governments and organizations worldwide. Striking the balance between innovation and safeguarding individual rights remains an ongoing challenge as these technologies continue to evolve and proliferate.
Future Trends
As research in computer vision and image recognition advances, the pursuit of more complex tasks has become a central focus. Scene understanding, for example, involves the ability to not only detect objects within an image but also to comprehend their spatial relationships and contextual significance. This capability has far-reaching applications, from autonomous navigation for robots and vehicles to enhancing the immersive quality of augmented reality experiences.
Furthermore, the evolution of computer vision extends into video analysis, enabling systems to process and interpret dynamic visual information. This has implications in fields like surveillance, where the tracking of objects and anomalies in real-time video feeds is critical for security.
Efforts to improve the robustness and fairness of AI image recognition systems are paramount. Researchers are actively working to address issues related to bias and discrimination, ensuring that these systems are equitable and do not perpetuate societal prejudices. This involves data curation, algorithmic transparency, and bias mitigation techniques.
Additionally, the integration of computer vision with other AI technologies, such as natural language processing (NLP), holds immense promise. This interdisciplinary approach allows AI systems to not only see and understand images but also to comprehend and generate human language, resulting in more comprehensive AI systems capable of multimodal interaction and understanding. Such systems have the potential to transform various industries, including customer service, healthcare, and content analysis. As these developments continue, the synergy between computer vision and other AI domains is poised to drive innovation and shape the future of artificial intelligence.
Computer vision and AI image recognition have indeed made remarkable progress since their early days, and their evolution shows no signs of slowing down. The ongoing advancements in these fields hold immense promise for a wide array of applications and carry significant societal implications.
In recent years, the fusion of deep learning techniques, particularly convolutional neural networks (CNNs), has pushed the boundaries of AI image recognition to new heights. This has led to astonishing levels of accuracy in tasks like object detection, facial recognition, and even natural language understanding from images. These developments have paved the way for innovations in industries such as healthcare, where AI assists in diagnosing medical conditions from scans, and in autonomous vehicles, where it enables vehicles to navigate and make decisions based on visual input.
However, with this progress comes the need for responsible development and ethical considerations. Concerns about privacy, bias, and the potential misuse of AI image recognition technologies have prompted discussions and regulations to ensure their safe and fair deployment.
Looking ahead, computer vision and AI image recognition are poised to continue reshaping our world, with applications ranging from environmental monitoring to creative arts. The journey of these technologies remains an exciting and transformative one, with countless possibilities yet to be explored.